TRUsted Framework for Federated LEarning Systems
We are online!
About the TRUFFLES Project website.

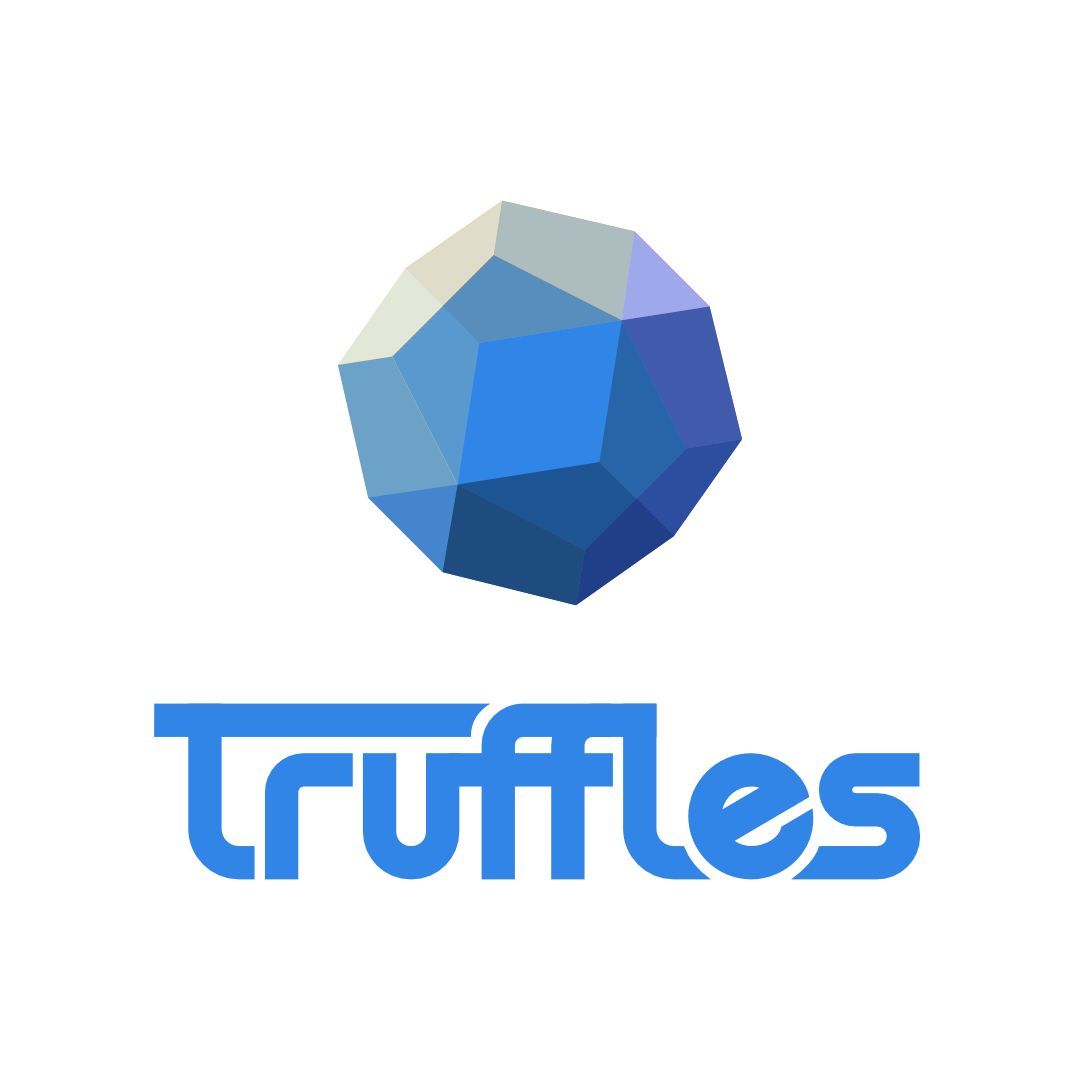

The TRUsted Framework for Federated LEarning Systems (TRUFFLES) project was granted in the strategic cybersecurity projects call by the INCIBE (Spain). It pursues research advances in the TRUSTED (DECENTRALIZED) FEDERATED LEARNING, (D)FL, area, that jointly addresses the challenges of privacy, security and robustness for distributed learning systems by combining information theory techniques, post-quantum encryption, crypto-coded computation and adversarial machine learning. Additionally, and since the INCIBE projects also have an important social aspect, TRUFFLES proposes some dissemination and social awareness activities.
Research Objectives
Evaluation of the level of privacy in FL
The aim is to define privacy metrics in FL that establish how much information an active adversary can obtain a priori (before running a given number of interactions of the learning algorithm) and a posteriori (after running that number of interactions). Real-time privacy loss estimation methods will be developed.
Design of secure aggregation techniques in (D)FL to prevent attacks against the aggregator(s) or the learning network computation nodes
Homomorphic encryption, secure multiparty computation and differential privacy techniques will be applied. Much of these techniques provide post-quantum resistance in a natural way, since they are either based on hard problems over lattices, or provide theoretical security under certain assumptions of non-collusion.
Analysis of threat models in FL
The aim is to identify realistic threats in FL environments where active adversaries try to infer as much information as possible during the learning process.
Design of attacks with active adversaries in FL
We intend to design membership inference attacks (to know if a subject belongs to a training group) and property inference attacks (to validate if a record in the database satisfies a property).
Evaluation of the level of privacy in FL
The aim is to define privacy metrics in FL that establish how much information an active adversary can obtain a priori (before running a given number of interactions of the learning algorithm) and a posteriori (after running that number of interactions). Real-time privacy loss estimation methods will be developed.
Design of secure aggregation techniques in (D)FL to prevent attacks against the aggregator(s) or the learning network computation nodes
Homomorphic encryption, secure multiparty computation and differential privacy techniques will be applied. Much of these techniques provide post-quantum resistance in a natural way, since they are either based on hard problems over lattices, or provide theoretical security under certain assumptions of non-collusion.
Design of authentication and accountability algorithms in (D)FL
This involves integrating Blockchain, authentication and direct model sharing technologies into a decentralized learning architecture to ensure traceability of learning processes.
Improving the efficiency and robustness of (D)FL algorithms to deal with adverse situations such as failures, interruption or delay in communications, and statistical inhomogeneity in the data
Improving the efficiency and robustness of (D)FL algorithms to deal with adverse situations such as failures, interruption or delay in communications, and statistical inhomogeneity in the data. The aim is to optimize the cost of communication and computation while maintaining the models in an adequate degree of updating depending on their availability and the conditions of connection and delay in the network.
Create a toolbox to experiment countermeasures in the private, secure and robust operation of (D)FL systems
This software will be used to generate a prototype (use case) in the finance field to detect fraudulent transactions.
O1
Analysis of threat models in FL
The aim is to identify realistic threats in FL environments where active adversaries try to infer as much information as possible during the learning process.
O2
Design of attacks with active adversaries in FL
We intend to design membership inference attacks (to know if a subject belongs to a training group) and property inference attacks (to validate if a record in the database satisfies a property).
O3
Evaluation of the level of privacy in FL
The aim is to define privacy metrics in FL that establish how much information an active adversary can obtain a priori (before running a given number of interactions of the learning algorithm) and a posteriori (after running that number of interactions). Real-time privacy loss estimation methods will be developed.
O4
Design of secure aggregation techniques in (D)FL to prevent attacks against the aggregator(s) or the learning network computation nodes
Homomorphic encryption, secure multiparty computation and differential privacy techniques will be applied. Much of these techniques provide post-quantum resistance in a natural way, since they are either based on hard problems over lattices, or provide theoretical security under certain assumptions of non-collusion.
O5
Design of authentication and accountability algorithms in (D)FL
This involves integrating Blockchain, authentication and direct model sharing technologies into a decentralized learning architecture to ensure traceability of learning processes.
O6
Improving the efficiency and robustness of (D)FL algorithms to deal with adverse situations such as failures, interruption or delay in communications, and statistical inhomogeneity in the data
Improving the efficiency and robustness of (D)FL algorithms to deal with adverse situations such as failures, interruption or delay in communications, and statistical inhomogeneity in the data. The aim is to optimize the cost of communication and computation while maintaining the models in an adequate degree of updating depending on their availability and the conditions of connection and delay in the network.
O7
Create a toolbox to experiment countermeasures in the private, secure and robust operation of (D)FL systems
This software will be used to generate a prototype (use case) in the finance field to detect fraudulent transactions.
Team
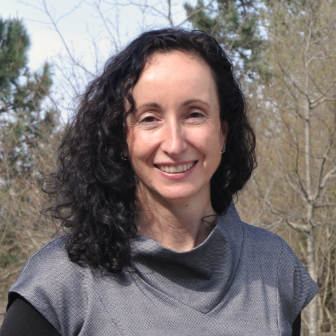
Rebeca P. Díaz Redondo
Principal Investigator
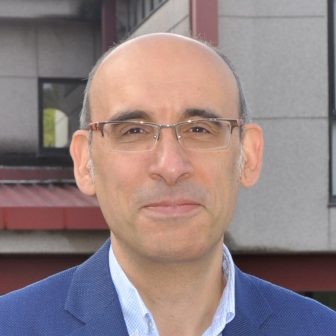
Fernando Pérez González
Principal Investigator
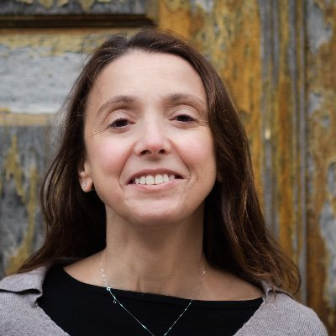
Ana Fernández Vilas
Researcher

Manuel Fernández Veiga
Researcher

Alberto Pedrouzo Ulloa
Researcher
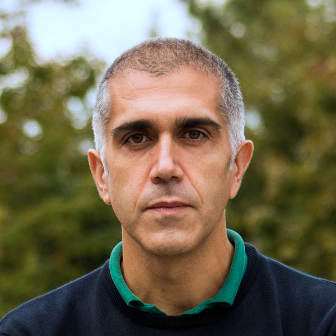
Pedro Comesaña Alfaro
Researcher
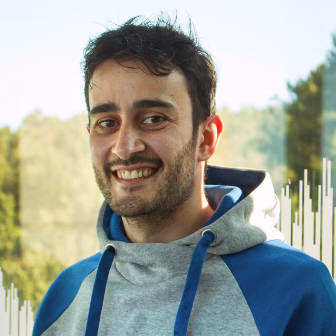
David Vázquez Padín
Researcher
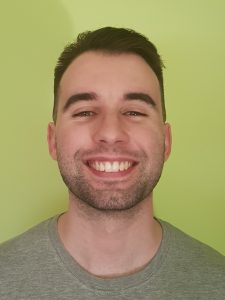
Carlos Beis Penedo
Researcher
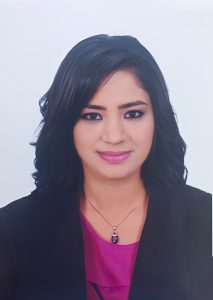
Rana Ibrahim
Researcher
